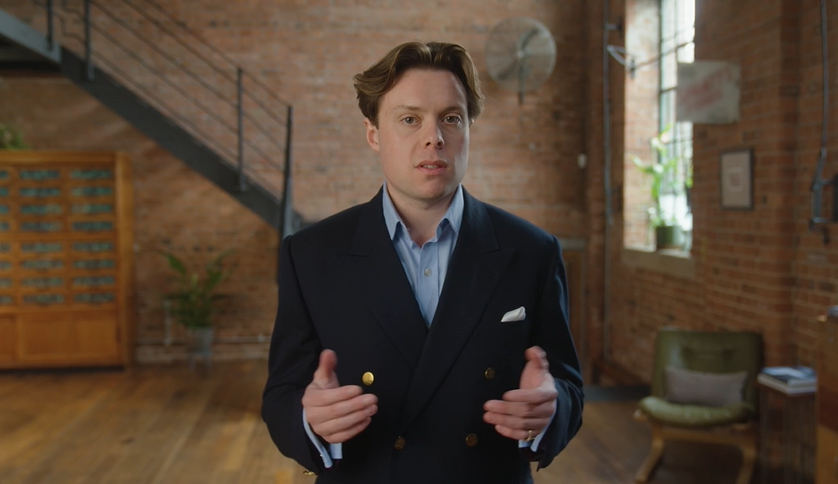
How did technology — one of the most challenging domains in operational risk — come to embrace techniques that have no scientific validity by way of effective risk measurement? Why did it bypass robust, accurate models, which existed decades before? How can organizations extract value from uncertainty?
The reality is consultative practices of the 20th century’s later years deceived the profession with a simple, easy-to-digest methodology for assessing risk. This took root in the embryonic stages of technology’s swift evolution. Readily embraced by established industry authorities (ISO, NIST, COSO etc.), the approach fast-became technology risk management lore, as RAG colors and qualitative scores (masquerading as numerics) populated lengthy management PowerPoints and ballooning risk registers.
Ironically, this post-dated the development of effective stochastic models by decades, particularly Monte Carlo Analysis, which empowered analysts to simulate complex scenarios under uncertainty. Yet the value-add of modelling a risk and its effects thousands of times over sadly failed to gain purchase against the simplicity of the risk matrix.
Rather than focus on assessing probabilities and expressing uncertainty, qualitative frameworks (although intended for risk-based decision making) peddled unchecked intuition as a method to predict fixed outcomes. The approach is inherently flawed because our base mental model cannot be relied upon, at least when left untrained and unmeasured. As Tversky and Kahneman showed as far back as the mid-70s, humans exist at the mercy of heuristics and cognitive biases, which affect our ability to make accurate forecasts on intuition alone.
This is where the matrix causes the greatest damage; its colorful formality and structure increases the perception of confidence in estimates. But this leads to diminishing returns — analysts feel more secure in their estimates but simultaneously drift further from reflecting reality. This ‘analysis placebo’ reinforces biases and facilitates other interfering components, including partition dependence and lie factors. Such models requiring subjective, fixed claims at a single point in time violate every fundamental tenet of risk.
Determining technology (or any) risk relies upon the expression of uncertainty. It’s an uncertain event with a given timeframe that — if it happens — will affect business objectives and decisions.
Uncertainty manifests in the form of random variables, which influence the scenarios we’re modelling — for example, an organization could experience a Citrix outage for three hours multiples times over an annual period, and the loss for each scenario would differ. This is a consequence of shifting internal and external factors that affect the loss incurred. The challenge for analysts is to reduce uncertainty through modelling to determine the extent of loss and inform decision making.
A necessary step-change in achieving uncertainty reduction is to estimate using probabilities. Probabilities help express the chance of discrete (e.g. event frequency) and continuous (e.g. productivity loss within a given range) variables manifesting in a given scenario: how often is this bad event likely to occur, and how much damage will we incur?
Analysts can use confidence intervals when estimating a range of plausible values (e.g. 90% Confidence Interval for inadvertent data loss costing between $15,000 and $450,000), expressing both their degree of surety, as well as uncertainty, for a given scenario. This structure provides a robust feedback model; it enables analysts to absorb updated information over time to refine their estimate. As analysts become calibrated (accurate in their estimates), they gain accumulative value through demonstrating increasing improvement in successive estimates.
This value is particularly evident when estimating loss ranges, which can be categorized in two ways: primary and secondary. Primary losses are those incurred every time a type of incident occurs. For example:
- Productivity downtime
- Incident response costs
- People, process and or technology costs in the fallout of an incident
Secondary losses are those incurred only certain times given a type of incident. For example:
- Stock price devaluation
- Regulatory penalties
- Revenue loss due to lost clients (existing or prospective)
The ability to estimate loss ranges in financial terms whilst simultaneously reflecting the analyst’s uncertainty is critical to delivering robust forecasting.
Although technology as a profession remains slow to adopt more advanced practices to risk measurement, focusing on reflecting uncertainty and capturing the variables influencing loss exposure is a critical step on the path to providing accurate, testable and repeatable forecasting, critical for directing effective decision making and investment.
In the How to Build a Quantitative Technology Risk Management System video, learn how to shift focus from a traditional qualitative approach to a more effective quantitative approach when it comes to successfully navigating an evolving risk landscape.
Ash Hunt
Ash Hunt is a global CISO, international keynote speaker and frequent board advisor with a decade of experience in complex, multinational environments. He has worked extensively across UK government departments, FTSE/FORBES organizations and Critical National Infrastructure (CNI), in addition to authoring the UK’s first quantitative framework and actuarial model for information risk. He has also served as a media commentator for Sky News and ITV on cyber security issues.